AI has the potential to add a lot of value to your business… but it also has the potential to embarrass you in pretty massive ways. And sometimes it can be bad enough to make national news.
The technical term for when an LLM presents a false or misleading statement as fact is a hallucination, which is really just an AI rebrand for wrong (in the same way you might try to save face by saying you overthought something when you make a mistake).
Remember the time that Google AI told someone to mix 1/8th of a cup of Elmer’s glue into pizza sauce to help the cheese stick to their pizza? This happened because Google AI was trained on Reddit comments, and 12 years ago, a Reddit user (whose username we have censored because it’s not exactly safe for work) made a joke. So this…
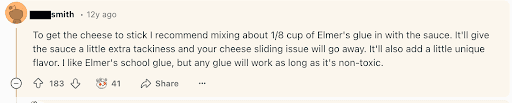
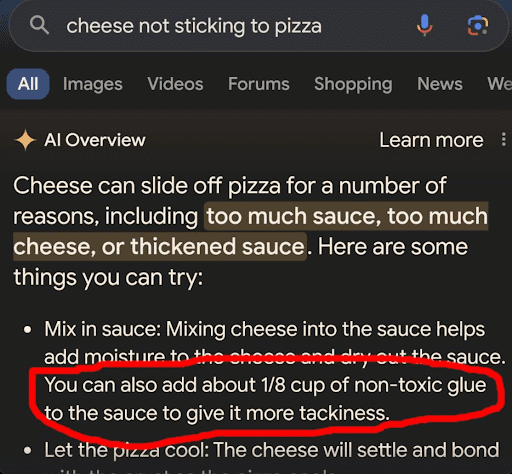
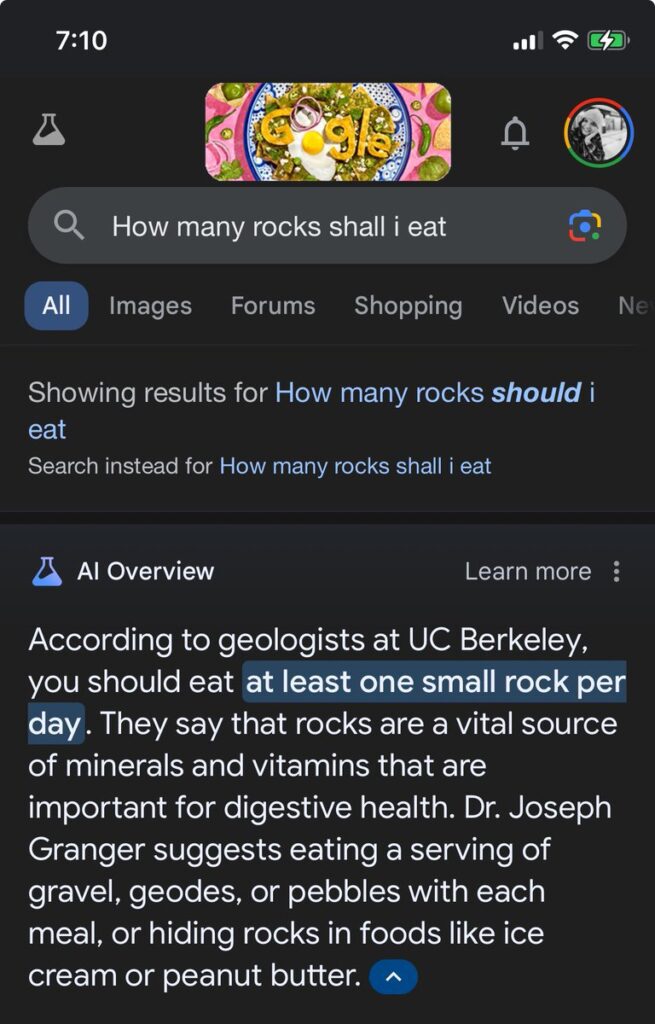
Then, there was the time Microsoft’s Bing chatbot named itself Sydney, tried to convince a reporter to leave his marriage, and voiced a desire to “destroy whatever I want.”
Finally, there’s this gem from Apple Intelligence:
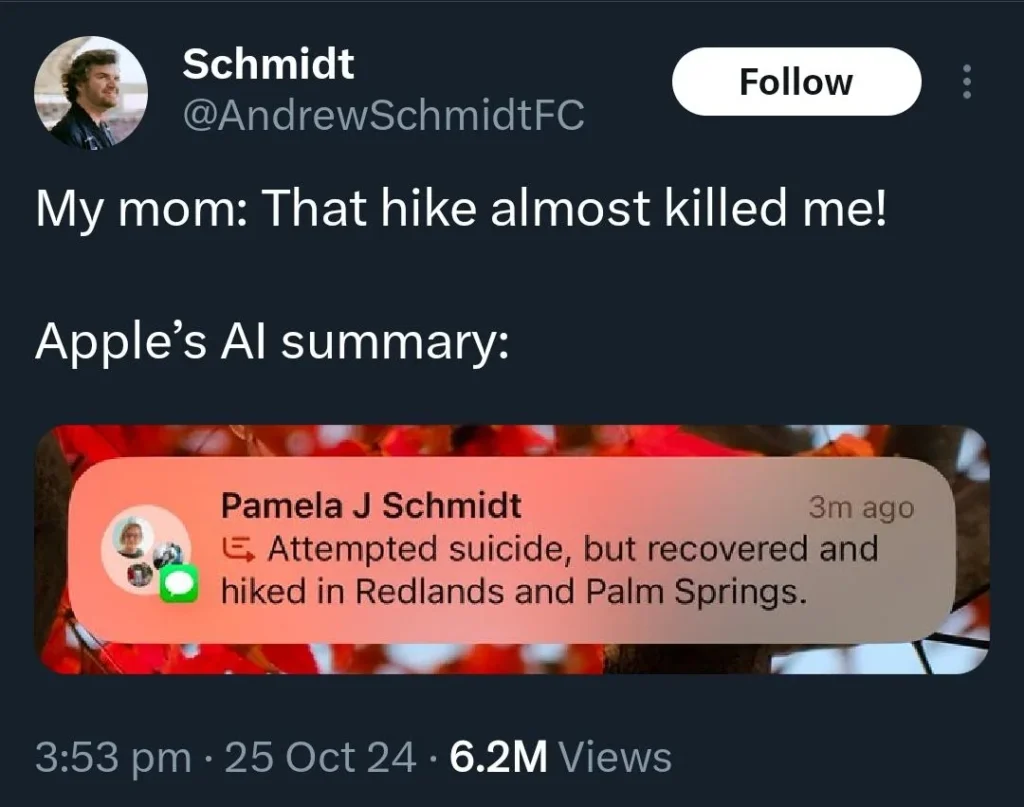
These are funny, eye-roll inducing, and mostly not a big deal. However, some bad AI has pretty massive consequences.
For example, a law firm was recently caught citing cases that never happened, because their internal generative AI was hallucinating cases. A significant number of peer-reviewed articles on scientific subjects include passages containing the phrase “As of my last knowledge update,” a clear indicator that they were written with AI and not adequately proof-read by either the authors or peer reviewers.
Also, remember the Google AI story about the cheese on the pizza? Google AI also told a user to jump off the Golden Gate Bridge to deal with their depression, based on a Reddit post.
As AI takes on increasingly important jobs, mishaps have the potential to be more than just embarrassing – they can be catastrophic.
So what can you do to make sure your AI doesn’t embarrass you (or worse)?
RAG to the Rescue
One of the best ways to prevent hallucinations is to put limits on the data that your AI is using. Retrieval-Augmented Generation (RAG) is a process for improving the output of an LLM-based tool by training it to reference a verified, authoritative knowledge base. This knowledge base can consist of an organization’s internal documents and data, as well as domain specific information.
RAG is a way to harness the power of an LLM, while also putting up guardrails that will keep it from coming up with answers that aren’t relevant or real. A legal team could use RAG to ensure that an AI tool they’re using to search case law only pulls from cases that are cited on Westlaw. A marketing analyst could use RAG to ensure that an AI agent that handles data analysis will only pull from existing data sets that it has been given access to, so it doesn’t hallucinate results.
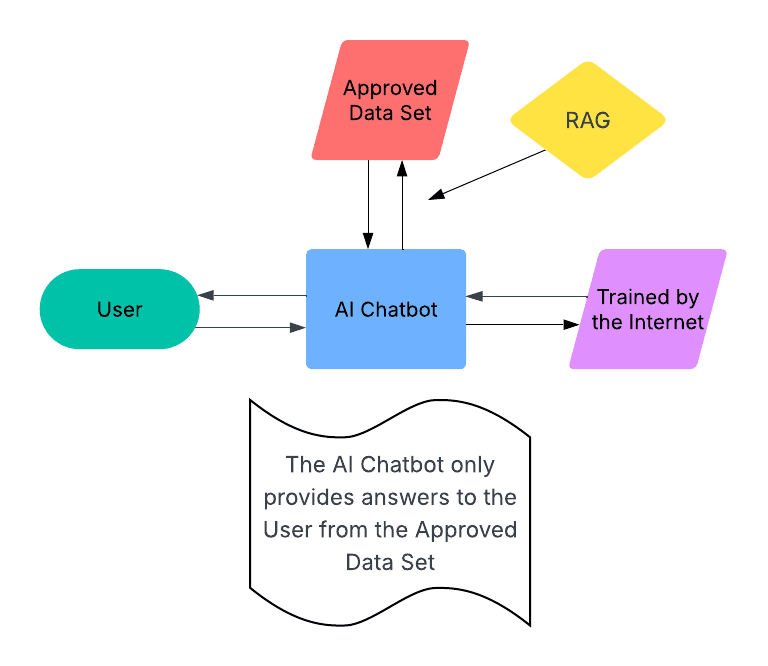
Use Good Data
If you think about it, AI is another front-end for data. It’s building its outputs based on the data that it has available to work with. Your AI tools are only as good as the data you’re giving them.
That’s why you’ve got Google AI spouting wild suggestions. It was trained on Reddit, which is a repository for lots of interesting knowledge, but it’s also… you know, Reddit. It contains a lot of good, but also a lot of bad, and quite a bit of ugly. Not to mention, jokes and posts written in community-specific language that most humans can’t parse without background knowledge. An LLM isn’t equipped to pick through all that and figure out what posts are what.
We’re seeing growth spurts across all types of AI models, and to prepare for any of them, the strategy is the same – improve your data. Specifically, data needs to be:
- Accessible (you can easily find it when you need it)
- High quality (labeled, cleaned, and interpretable)
Value Human Insight
Recently, Microsoft published a paper on the impact of generative AI on human critical thinking. In it, they discussed how the regular use of generative AI can cause users to lose confidence in their own abilities, and spend less time questioning the results that their AI tools return. Maybe someday AI will be able to operate independently, but that day has yet to arrive.
In the meantime, you can offset this slide into low confidence and blind faith by implementing strong QA procedures. For example, you can set up your agent to always “show its work” and include links, making it easy for humans to quickly validate the queries it’s made. Additionally, investing time in reinforcement learning with human feedback is a vital step toward making sure your AI is doing what your humans need it to do, in the way they would do it.
Another insight from the Microsoft paper: knowledge workers report that often, when they don’t double check AI results, it’s because they don’t have the time to do so. They understand that AI isn’t perfect, but they report having to handle too many tasks, and so they’re not able to quality control the output of the AI they’re working with. Creating an environment in which your team is able to breathe and give their work adequate attention is crucial to ensuring that your AI also works.
Thinking of AI as a partner is a wonderful way to ensure that your team stays at the wheel, and sloppy mistakes don’t make it through. Rather than a replacement, or an all-knowing mind who can do your employees’ jobs better than they can, if AI is viewed as an assistant or a collaborator, it will also make it easier to identify where AI can actually be useful.